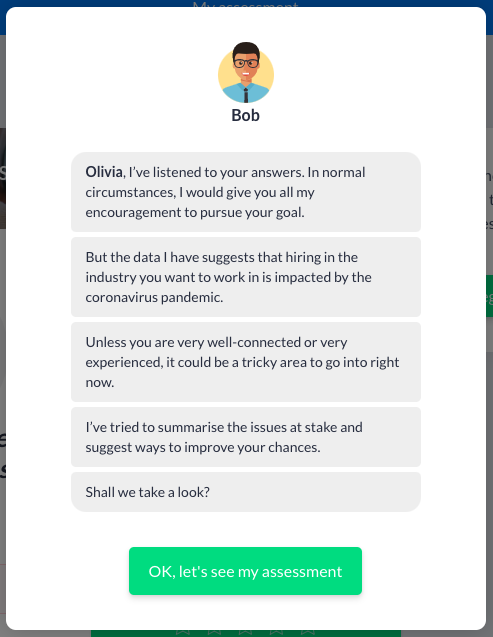
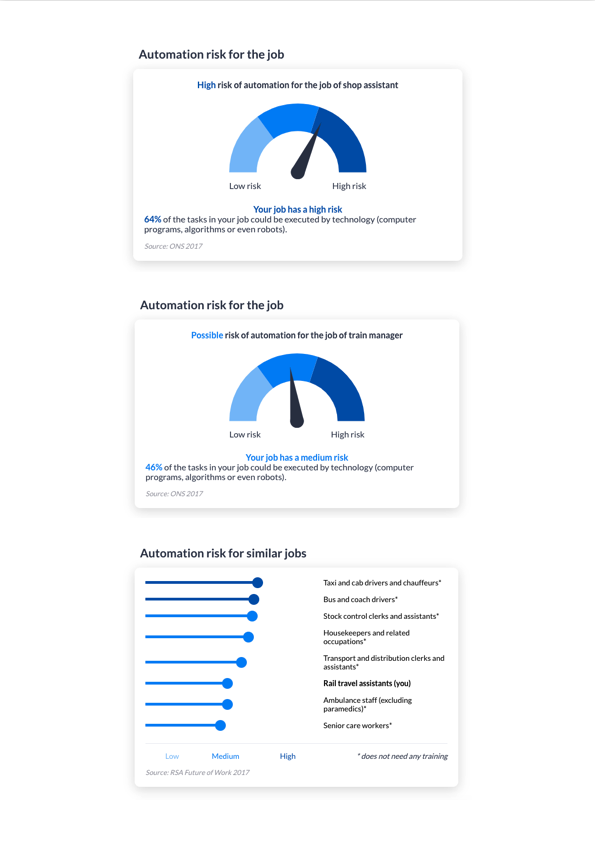
News – Blog
28 June 2021
Authors:
Hazel Klenk & Fay Sadro – the Learning and Work Institute
Jack Orlik, Amy Solder, Rhys Herriott and Sarah Mcloughlin – the CareerTech Challenge team.
A new report from Learning and Work Institute, Nesta and Challenge Works highlights the complexities (and opportunities) of using labour market data to support adults plan their careers.
Download the full report from Nesta’s website (PDF). If you are interested in discussing the findings from the report please contact us at [email protected].
The Skills for Jobs white paper has become a strategic centrepiece for UK government. In this white paper, the Department for Education sets out a plan to align the supply and demand for skills in the job market through a mix of measures that includes employer-led Local Skills Improvement Plans, new financial entitlements for training and ‘great careers support’.
If these approaches work, they could help bring the UK’s high level of ‘skills mismatch’ to best practice levels, boosting productivity by up to 5 per cent (OECD). They could also accelerate recovery from COVID-19 by giving workers the support they need to find new roles that realise their potential, make use of their skills and offer them a more secure future in a labour market that is being disrupted by new technology.
But how can ‘great careers support’ be delivered at a scale that has a real impact on the labour market? This was a central question that drove the CareerTech Challenge, a partnership between Nesta, Challenge Works and the Department for Education which sought to stimulate the development of new tools for career navigation, and uncover ‘what works’ to help adults develop new skills and find good work.
Of the 31 innovators supported by the CareerTech Challenge, 20 Prize finalists worked on building and testing new tools to make careers information, advice and guidance more accessible and tailored to the supply and demand for skills in local job markets. Their progress was documented and analysed through interviews by our evaluation partners, Learning and Work Institute providing a unique opportunity to understand the enablers and barriers for the development of ‘great careers support’. The research revealed an abundance of creativity among the innovators, and demonstrated the value of user testing, co-creation and partnerships with local stakeholders, such as FE colleges and employers. Yet it also uncovered a major challenge that is holding back innovation in the field – limited and inaccessible labour market data.
The deficiencies in the UK’s labour market data are illustrated by the experiences of the winners of the CareerTech Challenge Prize, the team developing Bob UK, a tool designed to provide instant, online careers advice and job recommendations based on information about local vacancies and the jobseeker’s skills. The developers attempted to source UK data that directly replicated data sources used to develop the version of Bob which has helped over 250,000 jobseekers in France. However, it became apparent that equivalent sources of data rarely existed. The Bob UK team was able to work around this issue by carefully combining alternative sources of data from a number of UK and non-UK sources.
Bob, the winning solution from the CareerTech Challenge Prize, curates a wide range of data to provide useful insights to jobseekers.
Many other innovators experienced similar barriers, finding that the publicly available data that could help people to make more informed decisions about their careers is often incomplete, difficult to use and poorly described. The impact of this is significant. A shocking insight from the report is that one solution enabled careers advisors to base course recommendations on labour market information for the first time. Prior to using this tool, such information was too time-consuming for careers advisors to uncover and analyse for it to be of use, and job seekers were given advice that was not based on employer demand for skills.
To address this issue of hidden and missing data and unleash the productivity-raising potential of better skills matching, the report makes a series of recommendations, including:
Nesta’ Data Analytics team has launched a number of initiatives that aim to fill gaps in the UK’s supply of labour market information:
As well as the CareerTech Challenge Prize, Challenge Works is continuing to support innovation which supports people adapt to a rapidly changing labour market: